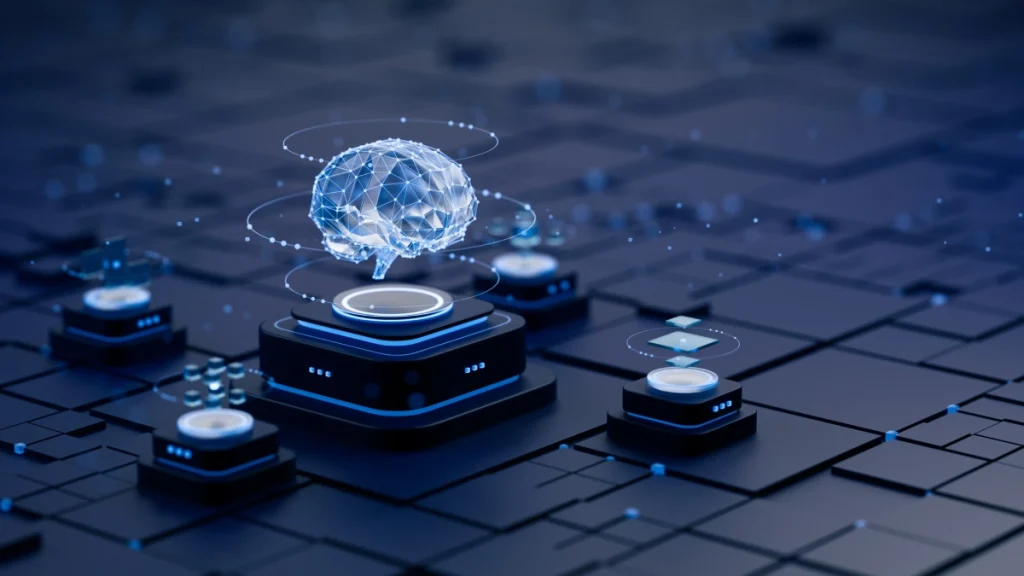
Over the last five years, we’ve seen a proliferation of proposed laws, voluntary principles and industry frameworks addressing the socially responsible use of AI.
Examples include the EU’s proposed AI Act, Singapore’s Model AI Governance Framework, Australia’s voluntary AI Ethics Principles and Microsoft’s Responsible AI Standard.
Terminology is varied at present, as stakeholders refer to either ethical AI, trustworthy AI or responsible AI; however they generally align on a set of common values which are clearly described in the European Commission’s Assessment List for Trustworthy AI:
- Human agency and oversight
- Technical robustness and safety
- Privacy and data governance
- Transparency
- Fairness
- Environmental and societal well-being; and
- Accountability.
All this activity at policy level has contributed to heightened public awareness of and interest in the responsible use of AI, as shown by recent criticism of Meta’s ad placement system, concerns over US predictive policing techniques, and investigation into Wesfarmers’ use of facial recognition technology in its Australian retail chains.
It is clear that in the near future developers and providers of AI will be required to demonstrate compliance with some form of values-based framework, and that’s a good thing. Here are 12 no-regrets steps we suggest for deploying AI responsibly, grouped according to the AI lifecycle.

Ideation
- Accountability If your organization is developing multiple AI-enabled products or tools, consider establishing an AI Review Board to approve use cases for new experiments and to review complaints or issues once AI-enabled products are launched. Involve leaders from different parts of the organisation, to increase breadth of perspective and sponsorship.
- Societal wellbeing The business case for each new project for AI-enabled products or services should consider their social and environmental risks and costs, as well as short term product success based on productivity and revenue.
Data Preparation
- Privacy Is your AI product trained on or does it use personal data? If so, check with your legal department: what privacy laws apply (such as the GDPR and/or Australia’s Privacy Act) and what must you do to comply?
- Data rights If your model uses third party datasets, make sure your use is consistent with any terms and conditions attached to the original data.
Model development
- Bias & Fairness Project risk registers should address the risk of potential negative discrimination (bias) against people on the basis of sex, race, colour, ethnicity, genetic features, language, religion or belief, political opinion, birth, disability, age or sexual orientation. Use technical tools to test and monitor for bias throughout development, deployment and use of the AI-enabled product.
- Transparency & Explainability Check that your developers are fully documenting the development process, i.e. the data and processes that produce the AI product’s output. Consider using some of the open source technical toolkits promoting explainability.
- Technical Robustness Talk to your developers about vulnerability to cyberattacks. Where possible, mitigate security risks at the model level (such as data poisoning, model evasion or model inversion) as well as the network and computing infrastructure level.
- Environmental wellbeing The environmental impact of AI is a big topic right now. Ask your developers if there are ways of reducing the carbon footprint of your AI product throughout its lifecycle, e.g. by using a pre-trained model as a starting point, selecting a different algorithm or using different computing hardware.
Deployment
- Human-centred Don’t forget human change management. Be clear about the role of humans in AI-enabled decision making and make sure human agents receive training on their roles and responsibilities before deploying an AI-enabled product.
- Transparency Communicate the technical limitations and potential risks of an AI-enabled product (such as its level of accuracy and/ or error rates) to the business owner and end-users. Consider using model cards to record information about the model.
Monitor and maintain
- Feedback loop Establish a process for third parties (e.g. suppliers, end-users, or data subjects) to report potential vulnerabilities, risks, or biases in the AI-enabled product to the product owner. Don’t reinvent the wheel, leverage existing processes – such as an HR complaints process or a customer support process – where possible.
- Monitor Do you have a mechanism to continually monitor your model performance and accuracy in production? There are several tools available in this space. Reach out to us at Red Marble AI if you would like to discuss your options!
Thanks for checking out our business articles. If you want to learn more, feel free to reach out to Red Marble AI. You can click on the "Let's Talk" button on our website or email Bronwyn, our Ai Govern expert at b.ross@redmarble.ai.
We appreciate your interest and look forward to sharing more with you!