Brief
- Hyper-Personalisation
- Prediction
- Conversation and Language
- Recognition
Downer Group is an integrated services company active in Australia and New Zealand. Listed on the Australian Securities Exchange and New Zealand Stock Exchange as Downer EDI, Downer is an ASX 200 company.
Challenge
Construction contracts often have clauses for varying the project timeline and costs due to unforeseen circumstances. If asbestos were to be found on site while excavating, this would trigger a contractual obligation to report the findings to the client in order to claim additional costs or a time extension. However, information relating to these claims are often only available in unstructured natural language site diary reports filled in by supervisors on site.
Solution
A process to digitise and capture supervisor input was put in place for ingestion by a custom language model that assigns to each site diary entry a set of flags indicating under which contractual clause an inferred event may be claimed.
Proven results in weeks, not years
Proven results in weeks, not years
Hold to view more details
Exec.
Briefing
2 Hours
Technology
Assessment
2-3 Days
Production
Trial
8-12 Weeks
AI Application
Deployment in Production
3-6 Months
Results
A reduction in supervisor workload, greater number of claimable events identified and vastly increased success rate of commercial claims due to the model’s ability to run batch analysis and operate in real-time.
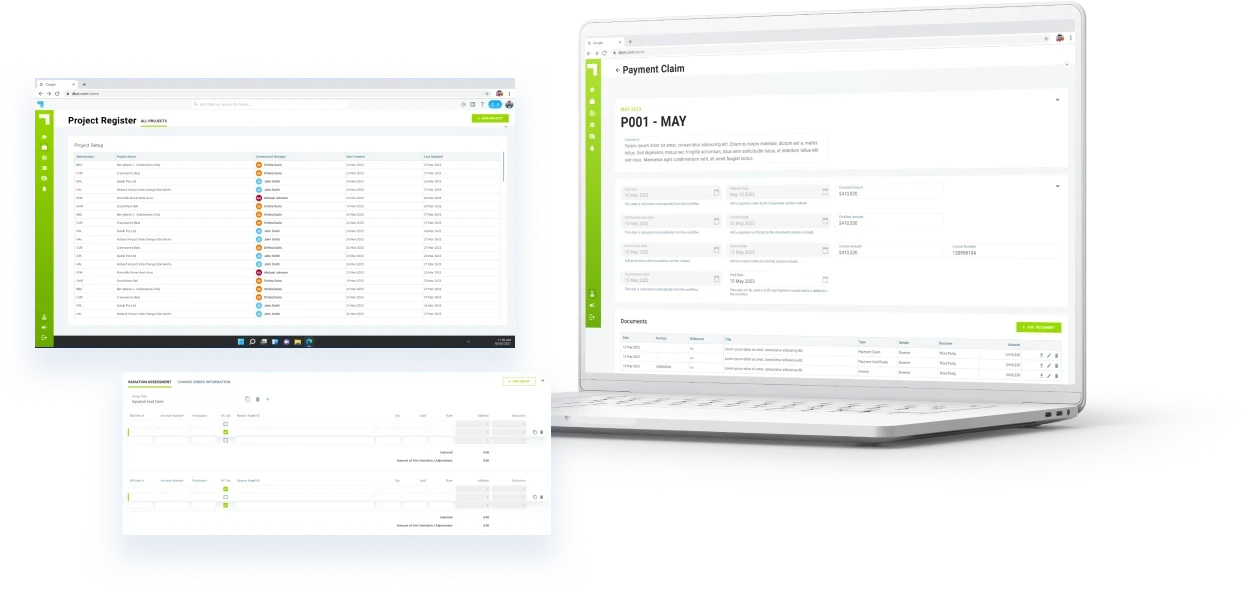
A reduction in supervisor workload, greater number of claimable events identified and vastly increased success rate of commercial claims…



Technical Approach
We began by investigating deep learning techniques to classify documents, namely, BERT, FastText, and ELMo, since we wanted to test if these pretrained models could bring sufficient semantic understanding to the construction language domain. Due to the nature of site diary entries following poor grammar and being littered with misspellings and unseen vocabulary, a simple keyword searching model outperformed these complex transformers.
We thus decided to develop a novel logic-based model. Essentially, for a given category, we interrogated the set of positively classified site diary entries to find complex word conjunctions that accounted for a large degree of the variance in predicting a positive classification, which we term positive facts. We then analysed the negatively classified set to find negating facts for each positive fact. A logic model was wrapped over the top to combine these learned understandings of the construction domain to classify each site entry.
Each fact represents an abstract, intuitive concept that the model has learned from the data.