Brief
- Hyper-Personalisation
- Prediction
- Conversation and Language
- Recognition
Webjet Limited is a global digital travel business operating in both consumer and wholesale markets. Webjet Limited’s Registered Office is located in Melbourne, Australia.
Challenge
The Online travel agent wanted to give compelling prices to its customers while maintaining revenue and growing market share. Discounting certain routes on certain days could achieve that but was labour intensive. Applying discounts dynamically could allow customers to obtain the best prices in the market.
Solution
They have a strong in-house technical team but lacked internal machine learning talent. Red Marble was engaged in a highly collaborative project, working closely with the business experts and the IT team to dynamically identify route combinations that are candidates for discounts to maintain competitive pricing while staying within the discounting budget.
Proven results in weeks, not years
Hold to view more details
Exec.
Briefing
2 Hours
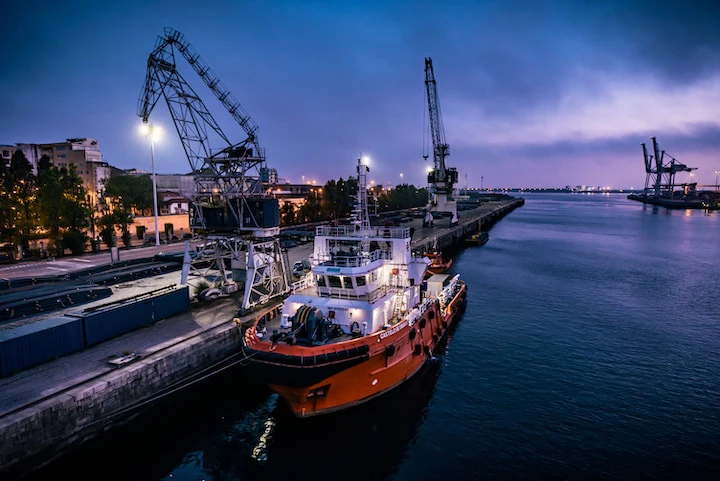
Technology
Assessment
2-3 Days
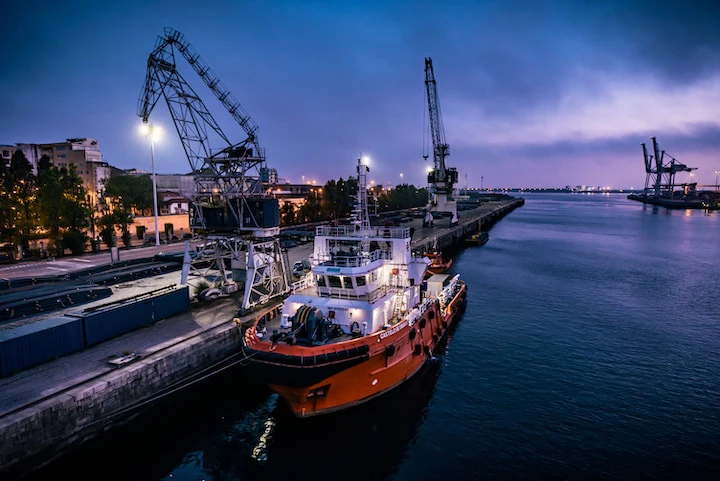
Production
Trial
8-12 Weeks
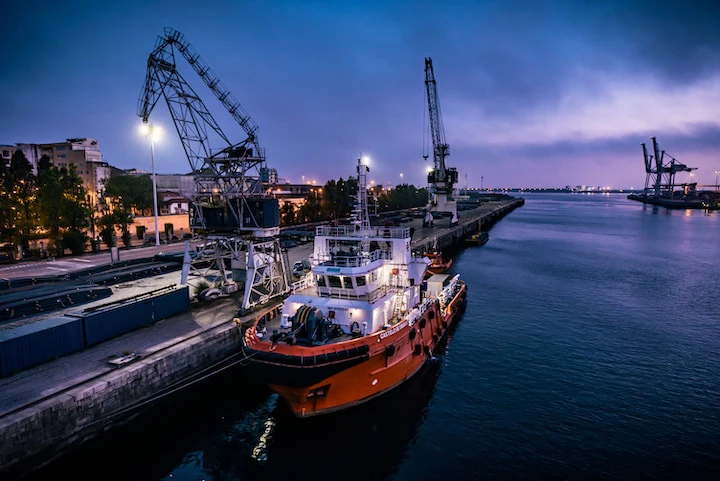
AI Application
Deployment in Production
3-6 Months
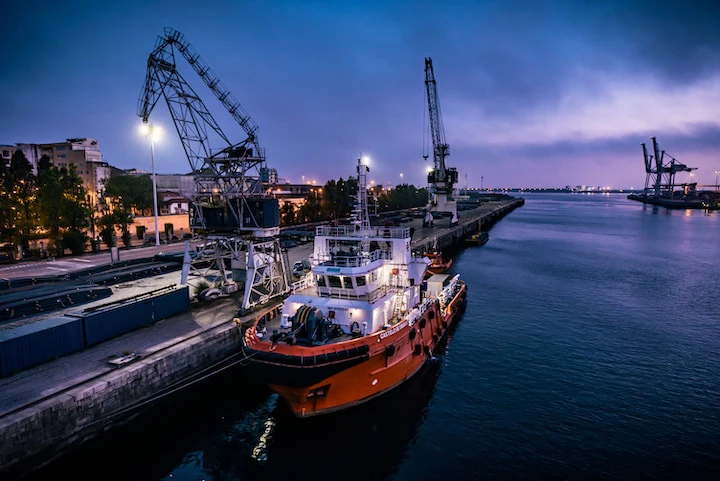
Results
Deployment of the AI-in-the-loop product resulted in better value for customers while increasing revenue and market share. The ability to configure the discount budget meant that spend could be accurately controlled.
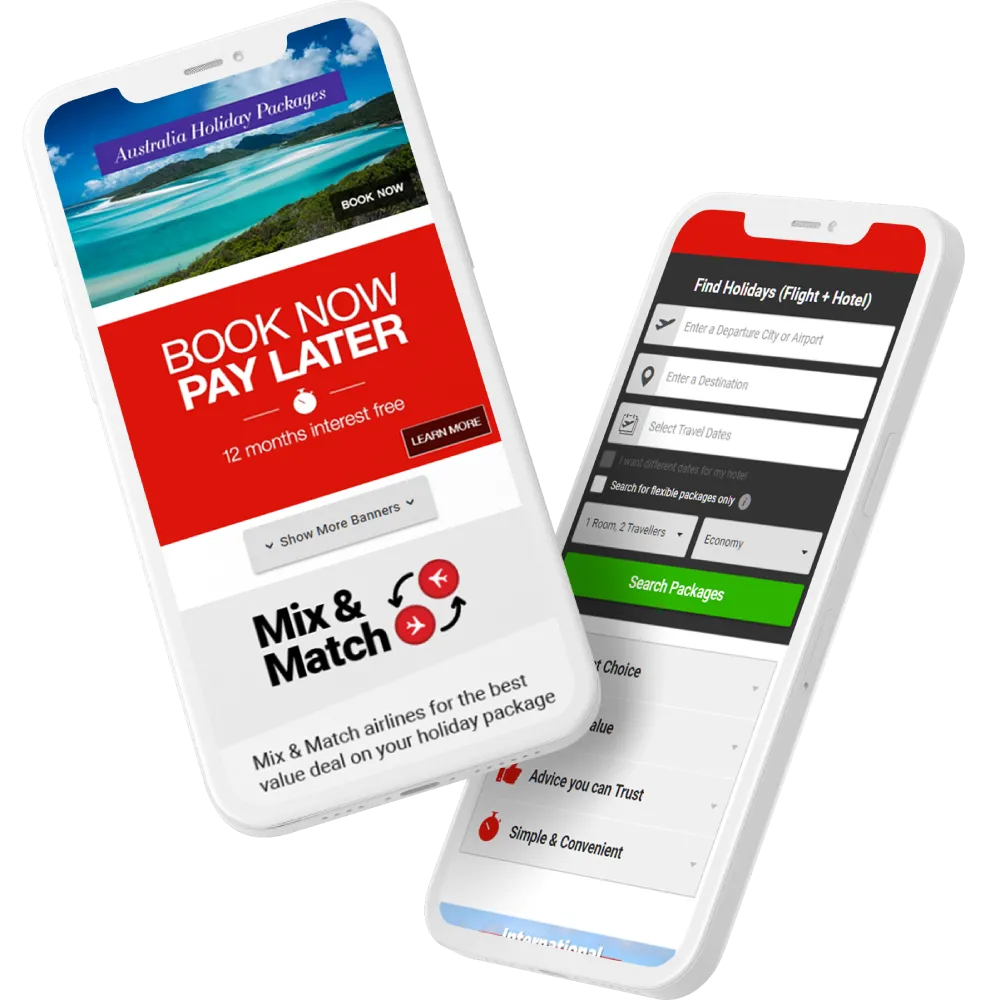
Deployment of the AI-in-the-loop product resulted in increased revenue and margins.
The ability to configure the discount budget meant that spend could be accurately controlled.
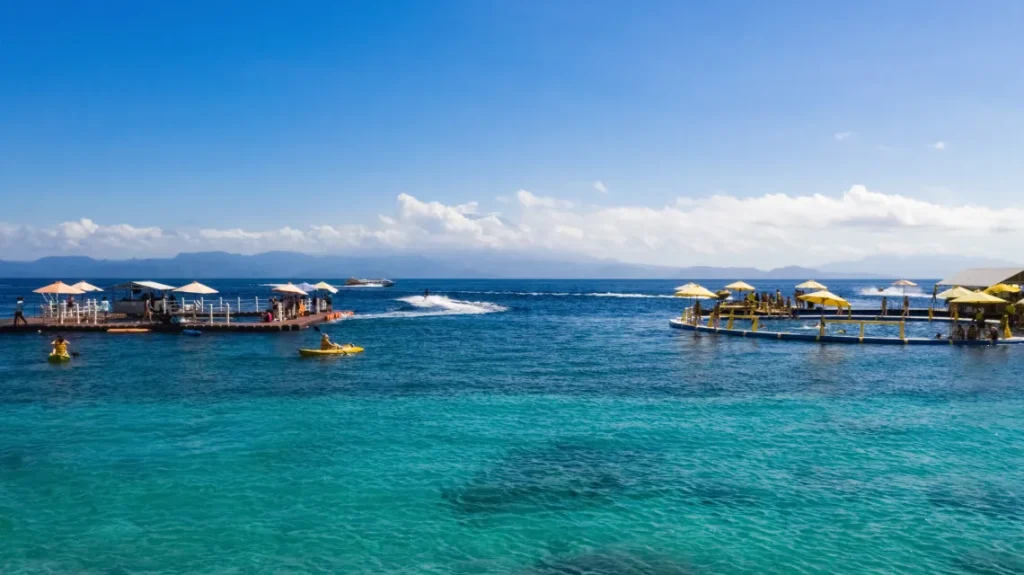
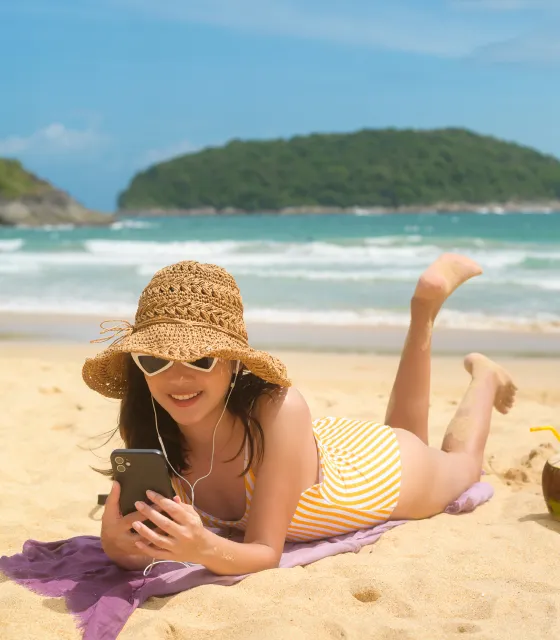
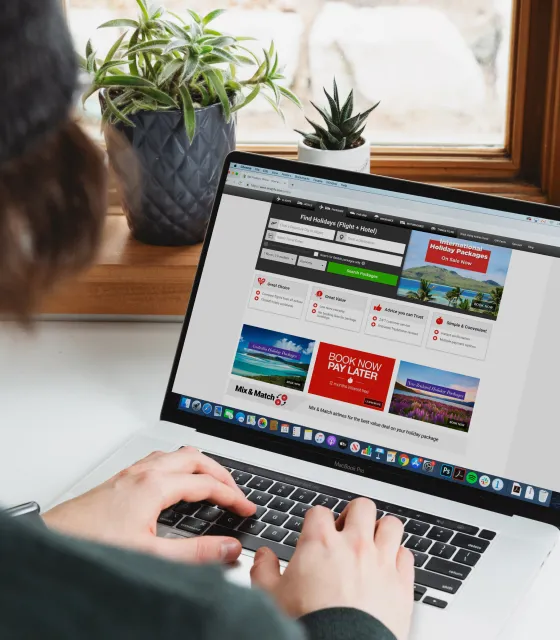
For the Techies
We initially performed business and data analysis to understand the existing discounting processes and the human reasoning that underpins the decisioning. We then created an ETL pipeline to feed a custom data model for machine learning. The data is used to forecast future supply and train a neural network that predicts the price sensitivities of each segment of customers.
The discount budget is then spread over the segments so as to achieve the required spend whilst attaining the maximum increase in revenue. One learning from this project relates to the way that macro-level changes in the environment – the Covid pandemic – can impact a tuned model and how to mitigate those risks.
We embedded our technical engineers with that of the customer’s and worked cooperatively to solve the problem, whilst providing full transparency of the collaborative process.